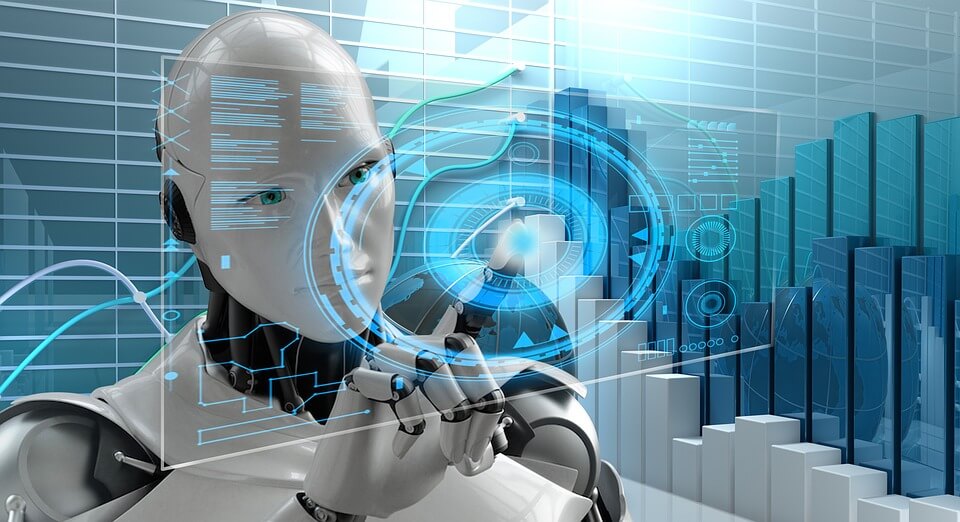
- January 8, 2020
- wladone3
- Business
Competition in retail banking may be more intense than ever as FinTechs and new market entrants fight with established players for deposits and market share. Retail banks that embrace advanced analytics and leverage their valuable data can gain a decisive competitive advantage.
1. Predicting client needs
Deeper client relationships are both more profitable and more loyal. By learning from their data, banks can identify, even anticipate, client needs that they can help with. Clients are far more likely to respond to a relevant offer and to have a favorable impression of your bank than they are if you are still sending indiscriminate offers with minuscule response rates.
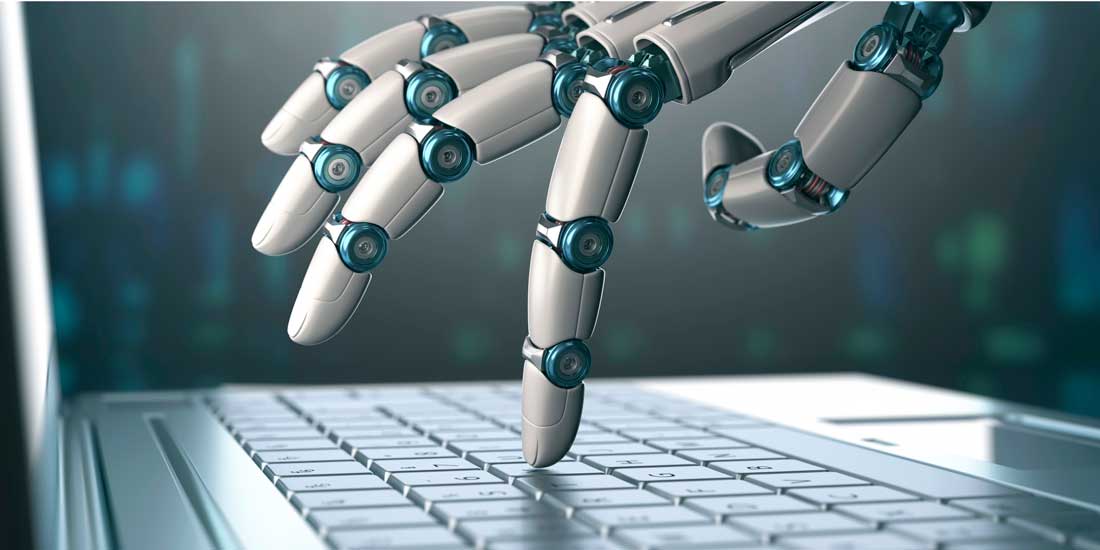
The most important use cases of Natural Language Processing are:
Document Summarization is a set of methods for creating short, meaningful descriptions of long texts (i.e. documents, research papers).
2. Keeping existing customers is at least as important as finding new ones.
Banks can learn from their client interaction data to identify customers at risk of attrition and take preemptive action. Even better, good models can identify the leading causes of attrition risk so that you can make process adjustments or improvements in order to hold onto more of your most valuable clients.
3. Price optimization and lifetime value
Many banks use a score-carding process in consumer lending, determining what terms to offer if the borrower meets certain criteria. Often, these are based on risk appetite rather than any insight into price elasticity or profit margin/volume tradeoffs. If banks knew which clients were likely to be the most profitable and knew how those clients were likely to respond to price differences, then they might price more aggressively in order to land those clients.
- Training data is used to train a model. It means that ML model sees that data and learns to detect patterns or determine which features are most important during prediction.
- Validation data is used for tuning model parameters and comparing different models in order to determine the best ones. The validation data should be different from the training data, and should not be used in the training phase. Otherwise, the model would overfit, and poorly generalize to the new (production) data.
- It may seem tedious, but there is always a third, final test set (also often called a hold-out). It is used once the final model is chosen to simulate the model’s behaviour on a completely unseen data, i.e. data points that weren’t used in building models or even in deciding which model to choose.
It’s not by any means exhaustive, but a good, light read prep before a meeting with an AI director or vendor – or a quick revisit before a job interview!
Aron Larsson
– CEO, Strategy Director
Lorem ipsum dolor sit amet, consectetur adipiscing elit, sed do eiusmod tempor incididunt ut labore et dolore magna aliqua. Ut enim ad minim veniam, quis nostrud exercitation ullamco laboris nisi ut aliquip ex ea commodo consequat. Duis aute irure dolor in reprehenderit in voluptate velit esse cillum dolore eu fugiat nulla pariatur.
Headings
- Artificial Intelligence (1)
- Business (4)
- Natural Language Processing (1)
- NLP (1)
- Technology (4)